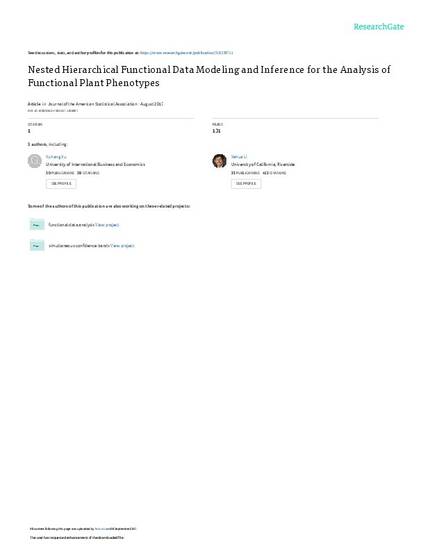
In a plant science Root Image Study, the process of seedling roots bending in response to gravity is recorded using digital cameras, and the bending rates are modeled as functional plant phenotype data. The functional phenotypes are collected from seeds representing a large variety of genotypes and have a three-level nested hierarchical structure, with seeds nested in groups nested in genotypes. The seeds are imaged on different days of the lunar cycle, and an important scientific question is whether there are lunar effects on root bending. We allow the mean function of the bending rate to depend on the lunar day and model the phenotypic variation between genotypes, groups of seeds imaged together, and individual seeds by hierarchical functional random effects. We estimate the covariance functions of the functional random effects by a fast penalized tensor product spline approach, perform multi-level functional principal component analysis (FPCA) using the best linear unbiased predictor of the principal component scores, and improve the efficiency of mean estimation by iterative decorrelation. We choose the number of principal components using a conditional Akaike information criterion and test the lunar day effect using generalized likelihood ratio test statistics based on the marginal and conditional likelihoods. We also propose a permutation procedure to evaluate the null distribution of the test statistics. Our simulation studies show that our model selection criterion selects the correct number of principal components with remarkably high frequency, and the likelihood-based tests based on FPCA have higher power than a test based on working independence. Supplementary materials for this article are available online.
Available at: http://works.bepress.com/dan-nettleton/121/
This is a manuscript of an article is published as Xu, Yuhang, Yehua Li, and Dan Nettleton. "Nested hierarchical functional data modeling and inference for the analysis of functional plant phenotypes." Journal of the American Statistical Association 113, no. 522 (2018): 593-606. doi: 10.1080/01621459.2017.1366907. Posted with permission.