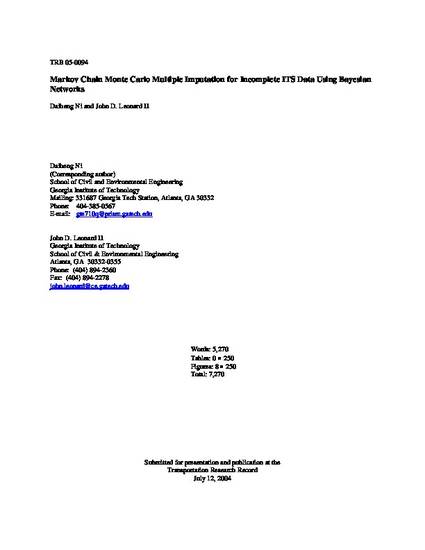
The rich ITS data is a precious resource for transportatio n researchers and practitioners. However, the usability of such resource is greatly limited by the issue of data missing. A lot of imputation methods have been proposed in the past decade. However, some issues ar e still not or not sufficiently addresse d. For example, the missing of entire records, temporal correlation in observations, natural char acteristics in raw data, and unbiased estimates for missing values. With these in mind, this paper proposes an advanced imputation method which is based on the recent development in other disciplines, especially applied statistics . It uses a Bayesian network to learn from the raw data and a Markov chain Monte Carlo technique to sample from the probability distributions learned by the Bayesian network. On the other hand, it imputes the missing data multiple times and makes statistical inference about the result. In addition, it incorporates a time series model so that it allows data missing in entire rows – an unfavorable missing pattern that is frequently seen in ITS data. Empirical study shows that the proposed method is robust and very accurate. It is ideal for use as a high- quality imputation method for off-line application.
- Bayesian networks,
- Markov chain Monte Carlo,
- Gibbs sampler,
- Data augmentation,
- Multiple imputation,
- Time series,
- Incomplete data,
- Intelligent transportation systems (ITS)
Available at: http://works.bepress.com/daiheng_ni/27/