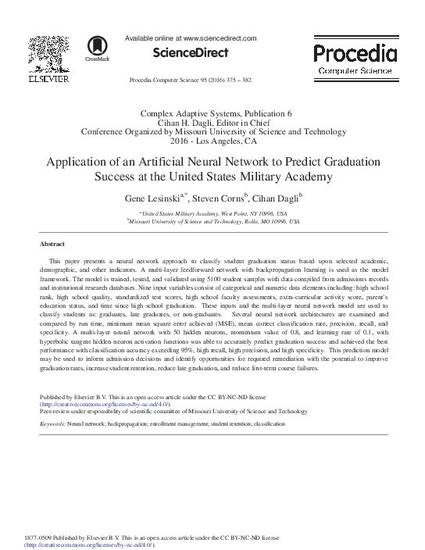
This paper presents a neural network approach to classify student graduation status based upon selected academic, demographic, and other indicators. A multi-layer feedforward network with backpropagation learning is used as the model framework. The model is trained, tested, and validated using 5100 student samples with data compiled from admissions records and institutional research databases. Nine input variables consist of categorical and numeric data elements including: high school rank, high school quality, standardized test scores, high school faculty assessments, extra-curricular activity score, parent's education status, and time since high school graduation. These inputs and the multi-layer neural network model are used to classify students as: graduates, late graduates, or non-graduates. Several neural network architectures are examined and compared by run time, minimum mean square error achieved (MSE), mean correct classification rate, precision, recall, and specificity. A multi-layer neural network with 50 hidden neurons, momentum value of 0.8, and learning rate of 0.1, with hyperbolic tangent hidden neuron activation functions was able to accurately predict graduation success and achieved the best performance with classification accuracy exceeding 95%, high recall, high precision, and high specificity. This prediction model may be used to inform admission decisions and identify opportunities for required remediation with the potential to improve graduation rates, increase student retention, reduce late graduation, and reduce first-term course failures.
- Adaptive systems,
- Backpropagation,
- Classification (of information),
- Complex networks,
- Education computing,
- Forecasting,
- Hyperbolic functions,
- Mean square error,
- Network architecture,
- Network layers,
- Students,
- Teaching,
- Backpropagation learning,
- Classification accuracy,
- Classification rates,
- Enrollment management,
- Feed-forward network,
- Minimum mean square errors,
- Student retention,
- United states military academies,
- Neural networks,
- Backpropagation,
- Classification
Available at: http://works.bepress.com/cihan-dagli/154/