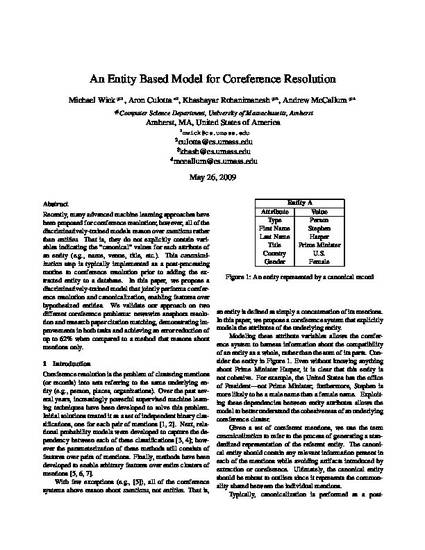
Unpublished Paper
An Entity Based Model for Coreference Resolution
(2009)
Abstract
Recently, many advanced machine learning approaches have been proposed for coreference resolution; however, all of the discriminatively-trained models reason over mentions, rather than entities. That is, they do not explicitly contain variables indicating the ``canonical'' values for each attribute of an entity (e.g., name, venue, title, etc.). This canonicalization step is typically implemented as a post-processing routine to coreference resolution prior to adding the extracted entity to a database. In this paper, we propose a discriminatively-trained model that jointly performs coreference resolution and canonicalization, enabling features over hypothesized entities. We validate our approach on two different coreference problems: newswire anaphora resolution and research paper citation matching, demonstrating improvements in both tasks and achieving an error reduction of up to 62% when compared to a method that reasons about mentions only.
Disciplines
Publication Date
2009
Comments
This is the pre-published version harvested from CIIR.
Citation Information
Michael Wick, Aron Culotta, Khashayar Rohanimanesh and Andrew McCallum. "An Entity Based Model for Coreference Resolution" (2009) Available at: http://works.bepress.com/andrew_mccallum/89/