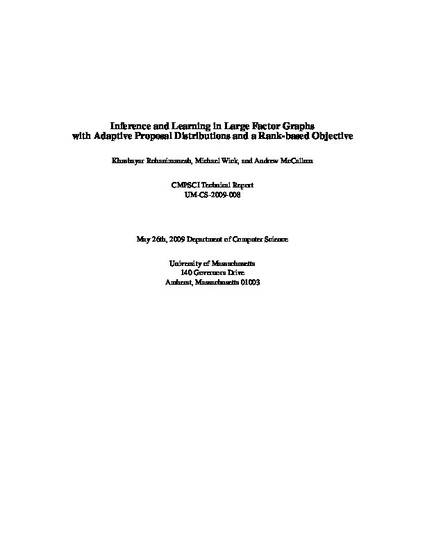
Unpublished Paper
Inference and Learning in Large Factor Graphs with Adaptive Proposal Distributions and a Rank-based Objective
(2009)
Abstract
Large templated factor graphs with complex structure that changes during inference have been shown to provide state-of-the-art experimental results in tasks such as identity uncertainty and information integration. However, inference and learning in these models is notoriously difficult. This paper formalizes, analyzes and proves convergence for the SampleRank algorithm, which learns extremely efficiently by calculating approximate parameter estimation gradients from each proposed MCMC jump. Next we present a parameterized, adaptive proposal distribution, which greatly increases the number of accepted jumps. We combine these methods in experiments on a real-world information extraction problem and demonstrate that the adaptive proposal distribution requires 27% fewer jumps than a more traditional proposer.
Disciplines
Publication Date
2009
Comments
This is the pre-published version harvested from CIIR.
Citation Information
Khashayar Rohanimanesh, Michael Wick and Andrew McCallum. "Inference and Learning in Large Factor Graphs with Adaptive Proposal Distributions and a Rank-based Objective" (2009) Available at: http://works.bepress.com/andrew_mccallum/86/