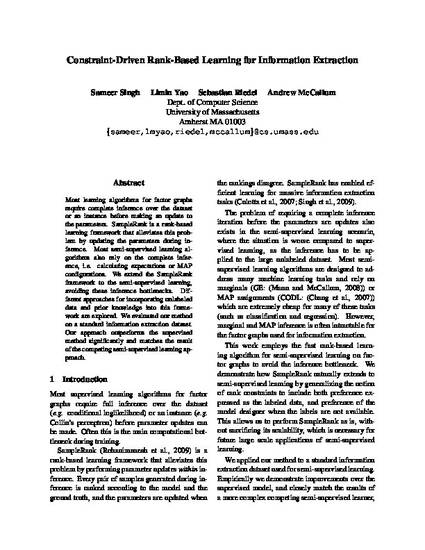
Unpublished Paper
Constraint-Driven Rank-Based Learning for Information Extraction
(2010)
Abstract
Most learning algorithms for factor graphs require complete inference over the dataset or an instance before making an update to the parameters. SampleRank is a rank-based learning framework that alleviates this problem by updating the parameters during inference. Most semi-supervised learning algorithms also rely on the complete inference, i.e. calculating expectations or MAP configurations. We extend the SampleRank framework to the semi-supervised learning, avoiding these inference bottlenecks. Different approaches for incorporating unlabeled data and prior knowledge into this framework are explored. We evaluated our method on a standard information extraction dataset. Our approach outperforms the supervised method significantly and matches the result of the competing semi-supervised learning approach.
Disciplines
Publication Date
2010
Comments
This is the pre-published version harvested from CIIR.
Citation Information
Sameer Singh, Limin Yao, Sebastian Riedel and Andrew McCallum. "Constraint-Driven Rank-Based Learning for Information Extraction" (2010) Available at: http://works.bepress.com/andrew_mccallum/76/