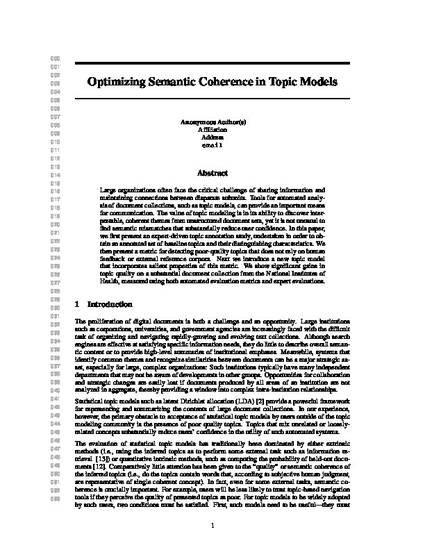
Unpublished Paper
Optimizing Semantic Coherence in Topic Models
(2010)
Abstract
Large organizations often face the critical challenge of sharing information and maintaining connections between disparate subunits. Tools for automated analysis of document collections, such as topic models, can provide an important means for communication. The value of topic modeling is in its ability to discover interpretable, coherent themes from unstructured document sets, yet it is not unusual to find semantic mismatches that substantially reduce user confidence. In this paper, we first present an expert-driven topic annotation study, undertaken in order to obtain an annotated set of baseline topics and their distinguishing characteristics. We then present a metric for detecting poor-quality topics that does not rely on human feedback or external reference corpora. Next we introduce a new topic model that incorporates salient properties of this metric. We show significant gains in topic quality on a substantial document collection from the National Institutes of Health, measured using both automated evaluation metrics and expert evaluations.
Disciplines
Publication Date
2010
Comments
This is the pre-published version harvested from CIIR.
Citation Information
D. Mimno, H. Wallach, E. Talley, M. Leenders, et al.. "Optimizing Semantic Coherence in Topic Models" (2010) Available at: http://works.bepress.com/andrew_mccallum/75/