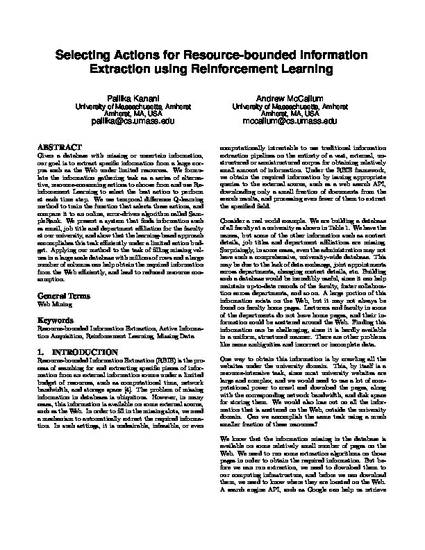
Unpublished Paper
Selecting Actions for Resource-bounded Information Extraction using Reinforcement Learning
(2011)
Abstract
Given a database with missing or uncertain information, our goal is to extract specific information from a large cor- pus such as the Web under limited resources. We formu- late the information gathering task as a series of alterna- tive, resource-consuming actions to choose from and use Re- inforcement Learning to select the best action to perform at each time step. We use temporal difference Q-learning method to train the function that selects these actions, and compare it to an online, error-driven algorithm called Sam- pleRank. We present a system that finds information such as email, job title and department affiliation for the faculty at our university, and show that the learning-based approach accomplishes this task efficiently under a limited action bud- get. Applying our method to the task of filling missing values in a large scale database with millions of rows and a large number of columns can help obtain the required information from the Web efficiently, and lead to reduced resource consumption.
Disciplines
Publication Date
2011
Comments
This is the pre-published version harvested from CIIR.
Citation Information
Andrew McCallum. "Selecting Actions for Resource-bounded Information Extraction using Reinforcement Learning" (2011) Available at: http://works.bepress.com/andrew_mccallum/68/