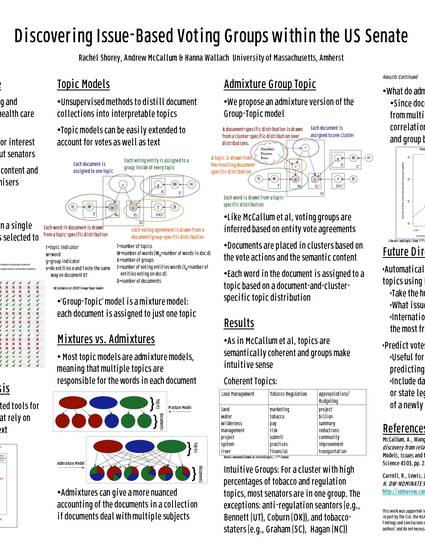
Presentation
Discovering Issue-Based Voting Groups within the US Senate
Women in Machine Learning Workshop (WiML)
(2010)
Abstract
Members of the US Senate cast votes on a wide array of issues. Understanding a senator's position on an issue is important to constituents, sources of campaign funding, and groups seeking to persuade senators or build consensus. Classifying senators' positions often falls into the hands of interest groups. Many lobbyists and issue-based organizations give senators scores based on the number of times senators vote in accordance with the organization's ideals. Organization staff must choose which bills to consider and then investigate their content manually. To produce more objective and replicable rankings, political scientists have developed statistical models to group and rank senators based on their voting records (Poole and Rosenthal, 1997, Clinton et al, 2004). These models group senators on the basis of voting records alone, accounting for bill content only in post hoc analysis or by selecting which bills to use as input. We propose a nonparametric Bayesian "group--topic" model for classification of senators. Following work by McCallum et al. (2008), we use the text of bills as well as roll call voting records to determine latent issue-based voting coalitions via an extension of latent Dirichlet allocation, in which each senator is assigned to a topic-specific voting group. McCallum et al.'s model is a mixture model -- that is, the model assumes each bill addresses only a single topic or issue. In contrast, our model is an admixture model, and therefore does not confine each bill to a single topic. In addition, our model makes use of a nonparametric Dirichlet Process prior to automatically determine the most appropriate number of latent groups and topics using the data. With this approach, it is no longer necessary to fix the number of latent groups and topics a priori. More importantly, allowing a variable number of groups provides valuable information about nuanced voting patterns on particular issues. These results may be helpful in determining which groups of senators are likely to support a particular bill or be willing to negotiate and compromise. By running our model on legislatures from multiple countries and examining the number and structure of the groups, we can also answer questions in comparative politics, such as which countries tend to exhibit particularly polarized legislatures.
Disciplines
Publication Date
December 6, 2010
Comments
This is the pre-published version harvested from CIIR.
Citation Information
Rachel Shorey, Andrew McCallum and Hanna Wallach. "Discovering Issue-Based Voting Groups within the US Senate" Women in Machine Learning Workshop (WiML) (2010) Available at: http://works.bepress.com/andrew_mccallum/66/