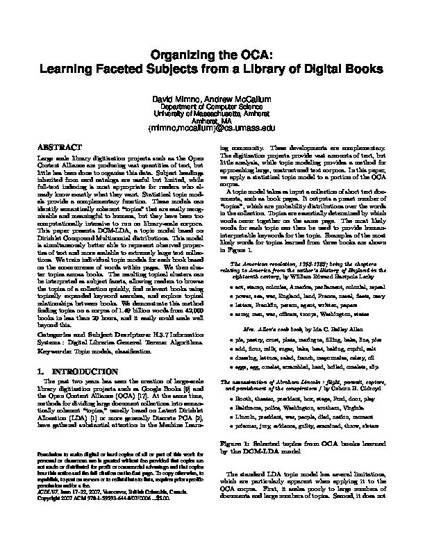
Unpublished Paper
Organizing the OCA: Learning Faceted Subjects from a Library of Digital Books
(2007)
Abstract
Large scale library digitization projects such as the Open Content Alliance are producing vast quantities of text, but little has been done to organize this data. Subject headings inherited from card catalogs are useful but limited, while full-text indexing is most appropriate for readers who already know exactly what they want. Statistical topic models provide a complementary function. These models can identify semantically coherent ``topics'' that are easily recognizable and meaningful to humans, but they have been too computationally intensive to run on library-scale corpora. This paper presents DCM-LDA, a topic model based on Dirichlet Compound Multinomial distributions. This model is simultaneously better able to represent observed properties of text and more scalable to extremely large text collections. We train the topic model using a form of stochastic EM. We begin by dividing the words in each book into topics independently of the other books. We then gather all the resulting topics and cluster them, learning Dirichlet parameters from each topic cluster. The resulting topical clusters can be interpreted as subject facets, allowing readers to browse the topics of a collection quickly, search for topic clusters using keywords, and explore topical relations between books. We demonstrate this method on 300 million words from 8000 books, and it easily could scale well beyond this.
Keywords
- Topic models,
- classification,
- Information Systems,
- Digital Libraries
Disciplines
Publication Date
2007
Comments
This is the pre-published version harvested from CIIR.
Citation Information
David Mimno and Andrew McCallum. "Organizing the OCA: Learning Faceted Subjects from a Library of Digital Books" (2007) Available at: http://works.bepress.com/andrew_mccallum/114/