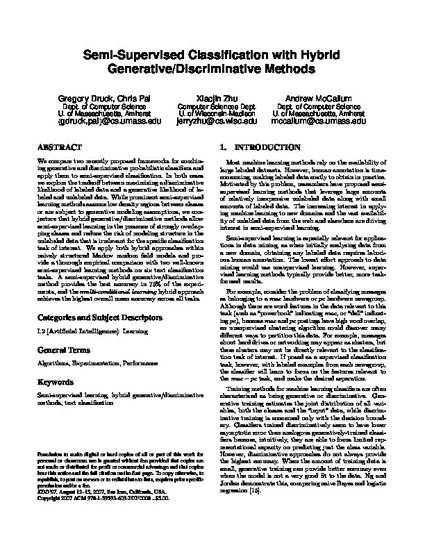
Unpublished Paper
Semi-Supervised Classification with Hybrid Generative/Discriminative Methods
(2007)
Abstract
In this paper, we study semi-supervised learning using hybrid generative/discriminative methods. Specifically, we compare two recently proposed frameworks for combining generative and discriminative classifiers and apply them to semi-supervised classification. In both cases we explore the tradeoff between maximizing a discriminative likelihood of labeled data and a generative likelihood of unlabeled data. While prominent semi-supervised learning methods assume low density regions between classes or are subject to generative modeling assumptions, hybrid generative/discriminative methods allow semi-supervised learning in the presence of strongly overlapping classes and reduce the risk of modeling structure in the unlabeled data that is irrelevant for the specific classification task of interest. We apply both approaches within naively structured Markov random field models and provide an extensive empirical comparison with other semi-supervised learning methods on several classification tasks. Hybrid/generative discriminative methods outperform other methods in 75% of the experiments.
Keywords
- Artificial Intelligence,
- Semi-supervised learning,
- hybrid generative/discriminative methods,
- text classification
Disciplines
Publication Date
2007
Comments
This is the pre-published version harvested from CIIR.
Citation Information
Gregory Druck, Chris Pal, Xiaoping Zhu and Andrew McCallum. "Semi-Supervised Classification with Hybrid Generative/Discriminative Methods" (2007) Available at: http://works.bepress.com/andrew_mccallum/100/