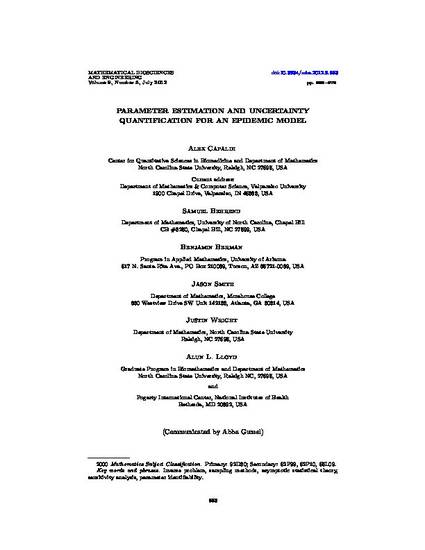
Article
Parameter Estimation and Uncertainty Quantication for an Epidemic Model
Mathematical Biosciences and Engineering
(2012)
Abstract
We examine estimation of the parameters of Susceptible-Infective-Recovered (SIR) models in the context of least squares. We review the use of asymptotic statistical theory and sensitivity analysis to obtain measures of uncertainty for estimates of the model parameters and the basic reproductive number (R0 )—an epidemiologically significant parameter grouping. We find that estimates of different parameters, such as the transmission parameter and recovery rate, are correlated, with the magnitude and sign of this correlation depending on the value of R0. Situations are highlighted in which this correlation allows R0 to be estimated with greater ease than its constituent parameters. Implications of correlation for parameter identifiability are discussed. Uncertainty estimates and sensitivity analysis are used to investigate how the frequency at which data is sampled affects the estimation process and how the accuracy and uncertainty of estimates improves as data is collected over the course of an outbreak. We assess the informativeness of individual data points in a given time series to determine when more frequent sampling (if possible) would prove to be most beneficial to the estimation process. This technique can be used to design data sampling schemes in more general contexts.
Keywords
- Inverse problem,
- sampling methods,
- asymptotic statistical theory,
- sensitivity analysis,
- parameter identifiability
Disciplines
Publication Date
July, 2012
Publisher Statement
doi:10.3934/mbe.2012.9.553
Citation Information
Alex Capaldi, Samuel Behrend, Benjamin Berman, Jason Smith, et al.. "Parameter Estimation and Uncertainty Quantication for an Epidemic Model" Mathematical Biosciences and Engineering Vol. 9 Iss. 3 (2012) Available at: http://works.bepress.com/alex_capaldi/1/